The use of artificial intelligence (AI) is exploding across many branches of science. Between 2012 and 2022, the average proportion of scientific papers engaging with AI, across 20 fields, quadrupled (see ‘AI’s rise in research’), including economics, geology, political science and psychology1.
Hopes are high that AI can accelerate scientific discovery, because the rate at which fundamental advances are made seems to be slowing down: despite there being more funding, publications and personnel, we are making advances at a slower pace.
But the rush to adopt AI has consequences. As its use proliferates — in forecasting disease outbreaks, predicting people’s life outcomes and anticipating civil wars — some degree of caution and introspection is warranted. Whereas statistical methods in general carry a risk of being used erroneously, AI carries even greater risks owing to its complexity and black-box nature. And errors are becoming increasingly common, especially when off-the-shelf tools are used by researchers who have limited expertise in computer science. It is easy for researchers to overestimate the predictive capabilities of an AI model, thereby creating the illusion of progress while stalling real advancements.
Here, we discuss the dangers and suggest a set of remedies. Establishing clear scientific guidelines on how to use these tools and techniques is urgent.
There are many ways in which AI can be deployed in science. It can be used to effectively comb through previous work, or to search a problem space (of, say, drug candidates) for a solution that can then be verified through conventional means.
Another use of AI is to build a machine-learning model of a phenomenon of interest, and interrogate it to gain knowledge about the world. Researchers call this machine-learning-based science; it can be seen as an upgrade of conventional statistical modelling. Machine-learning modelling is the chainsaw to the hand axe of statistics — powerful and more automated, but dangerous if used incorrectly.
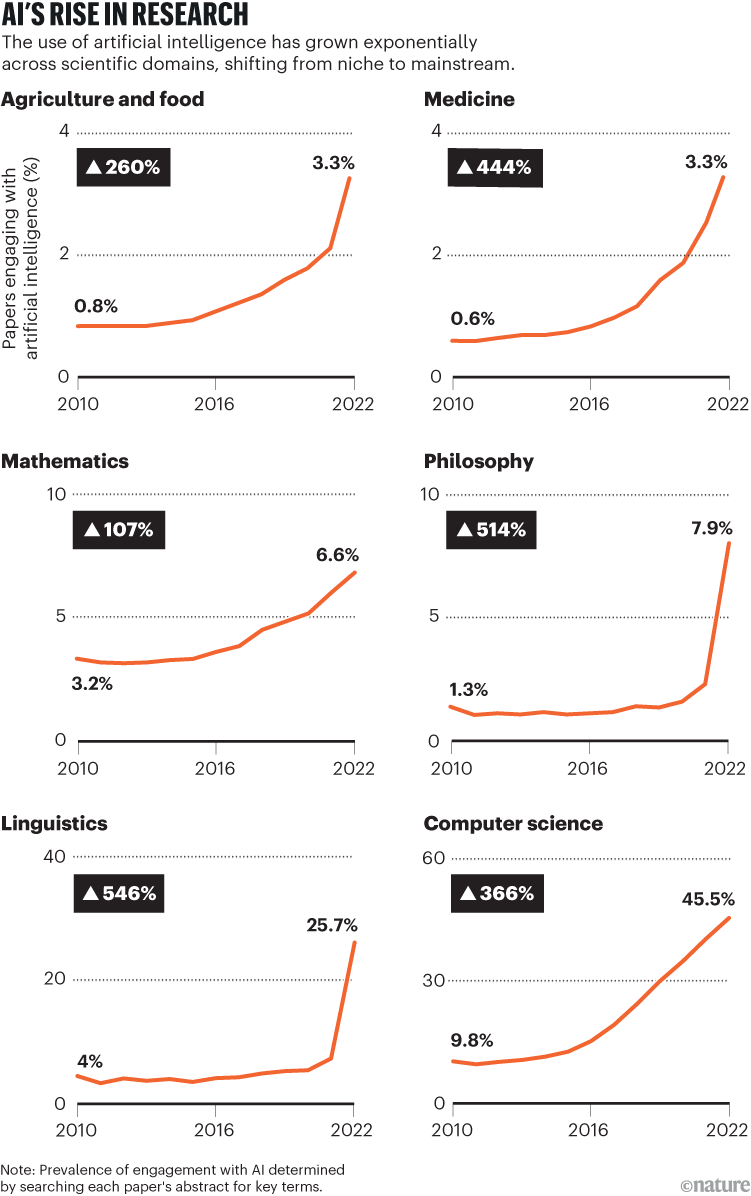
SOURCE: Ref. 1
Our concern is mainly about these modelling-based approaches, in which AI is used to make predictions or test hypotheses about how a system functions. One common source of error is ‘leakage’, an issue that arises when information from a model’s evaluation data improperly influences its training process. When this happens, a machine-learning model might simply memorize patterns in the evaluation data instead of capturing the meaningful patterns behind the phenomenon of interest. This limits the real-world applicability of such models and produces little in terms of scientific knowledge.
Through our investigations and compiling existing evidence, we have found that papers across at least 30 scientific fields — ranging from psychiatry and molecular biology to computer security — that use machine learning are plagued with leakage2 (see go.nature.com/4ieawbk). It is a type of ‘teaching to the test’, or, worse, giving the answers away before the exam.
For example, during the COVID-19 pandemic, hundreds of studies stated that AI could diagnose the disease using just chest X-rays or CT scans. A systematic review of 415 such studies found that only 62 met basic quality standards3. Even among them, flaws were widespread, including poor evaluation methods, duplicate data and lack of clarity on whether ‘positive’ cases were from people with a confirmed medical diagnosis.
In more than a dozen studies, the researchers had used a training data set in which all COVID-positive cases were in adults, and the negatives were in children aged between one and five. As a result, the AI model had merely learnt to distinguish between adults and children, but the researchers mistakenly concluded that they had developed a COVID-19 detector.
It’s hard to systematically catch errors such as these because evaluating predictive accuracy is notoriously tricky and, as yet, lacks standardization. Computer codebases can be thousands of lines long. Errors can be hard to spot, and a single error can be costly. Thus, we think that the reproducibility crisis in machine-learning-based science is still in its early days.
With some studies now using large language models in research — for instance, by using them as surrogates for human participants in psychological experiments — there are even more ways in which research might be irreproducible. These models are sensitive to inputs; small changes in the wording of prompts can cause notable changes to outputs. And because the models are often owned and operated by private companies, access to them can be restricted at any point, making such studies difficult to replicate.
Fooling ourselves
A greater risk from the hasty adoption of AI and machine learning lies in the fact that the torrent of findings, even if error-free, might not result in true scientific progress.
To understand that risk, consider the impact of an enormously influential paper from 2001, in which statistician Leo Breiman astutely described the cultural and methodological differences between the fields of statistics and machine learning4.
Will AI jeopardize science photography? There’s still time to create an ethical code of conduct
He strongly advocated the latter, including the adoption of machine-learning models over simpler statistical models, with predictive accuracy prioritized over questions of how faithfully the model represents nature. In our view, this advocacy did not mention the known limitations of the machine-learning approach. The paper doesn’t make enough of a distinction between the use of machine-learning models in engineering and in the natural sciences. Although Breiman found that such black-box models can work well in engineering, such as by classifying submarines using sonar data, they are harder to use in natural science, in which explaining nature (say, the principles behind the propagation of sound waves in water) is the whole point.
This confusion is still widespread, and too many researchers are seduced by the commercial success of AI. But just because a modelling approach is good for engineering, it doesn’t mean that it’s good for science.
There is an old maxim that ‘every model is wrong, but some models are useful’. It takes a lot of work to translate outputs from models to claims about the world. The toolbox of machine learning makes it easier to build models, but it doesn’t necessarily make it easier to extract knowledge about the world, and might well make it harder. As a result, we run the risk of producing more but understanding less5.
Science is not merely a collection of facts or findings. Actual scientific progress happens through theories, which explain a collection of findings, and paradigms, which are conceptual tools for understanding and investigating a domain. As we move from findings to theories to paradigms, things get more abstract, broader and less amenable to automation. We suspect that the rapid proliferation of scientific findings based on AI has not accelerated — and might even have inhibited — these higher levels of progress.
If researchers in a field are concerned about flaws in individual papers, we can measure their prevalence by analysing a sample of papers. But it is hard to find smoking-gun evidence that scientific communities as a whole are overemphasizing predictive accuracy at the expense of understanding, because it is not possible to access the counterfactual world. That said, historically, there have been many examples of fields getting stuck in a rut even as they excelled at producing individual findings. Among them are alchemy before chemistry, astronomy before the Copernican revolution and geology before plate tectonics.
A shout-out for AI studies that don’t make the headlines
The story of astronomy is particularly relevant to AI. The model of the Universe with Earth at its centre was extremely accurate at predicting planetary motions, because of tricks such as ‘epicycles’ — the assumption that planets move in circles whose centres revolve around Earth along a larger circular path. In fact, many modern planetarium projectors use this method to compute trajectories.
Today, AI excels at producing the equivalent of epicycles. All else being equal, being able to squeeze more predictive juice out of flawed theories and inadequate paradigms will help them to stick around for longer, impeding true scientific progress.
The paths forward
We have pointed out two main problems with the use of AI in science: flaws in individual studies and epistemological issues with the broad adoption of AI.